This conference will feature three keynote speakers.
Keynote Speaker 1: Professor Mark van der Laan, University of California, Berkeley
Topic: Addressing Multiple Testing in Causal Inference based on RWE Studies
Generating RWE in real world studies in a regulatory type setting is challenging from various perspectives. Firstly, the analysis needs to be pre-specified, maximally powerful, and provide valid coverage and type I error control. From this perspective, innovative designs, such as hybrid studies and adaptive designs, combined with a priori-specified tailored implementation of (double robust and efficient) TMLE incorporating state of the art machine learning through super-learning provides a rigorous approach for statistical inference w.r.t well defined target estimands. We provide a quick overview of some of these advances applied in our collaborations with FDA and industry. In combination with sensitivity analysis this can also result in causal inference for observational studies that allow for a causal gap between the statistical estimand and causal quantity of interest.
Another challenge is that one is often interested in many estimands such as causal effects on various secondary outcomes or within various subgroups so that multiple testing needs to be addressed. We discuss maximally powerful step-down multiple testing procedures based on achieving the desired type I error control (e.g. FWE, FDR, GFWE) under the multivariate mean zero normal limit distribution, or bootstrap approximations thereof, that take into account the often-strong correlation between the test statistics (and are therefore far more powerful than Bonferoni adjustments or methods based on marginal p-values only). These null distributions are always valid and thus not relying on a so-called subset pivotality condition. To make such procedures maximally robust it is important that the marginal distributions of the test statistics are well captured by the chosen null distribution. We discuss quantile-quantile function transformed joint null distributions that have controlled marginal null distributions such as a permutation distribution, while still estimating the joint correlation structure of the test-statistics. In addition, we discuss a method that control FDR while utilizing a screening algorithm that data adaptively reduces the set of null hypotheses.
We also present methods that avoid large scale multiple testing. Firstly, we present inference for data adaptive target estimands that require an a priori specified algorithm that generates one or more estimands of interest based on searching the space of candidate estimands, and subsequently applies a cross-fitted TMLE, or online-stabilized one-step estimator, to estimate it efficiently with valid inference. An important example is the counterfactual mean outcome under an estimate of the optimal individualized treatment rule among a set of candidate rules or all rules that use a user supplied set of variables. Other example is learning a most predictive (or strongest effect modifier of a treatment) SNP or gene among a large collection of variables. These methods provide valid and powerful inference without any multiple testing adjustment, at cost of possible interpretation due to the data adaptive estimand being an average across sample splits of sample split specific estimands. We discuss application of this approach for learning a best weighted combination of multiple clinical outcomes in rare disease regulatory studies, again, avoiding multiple testing adjustment (part of a FDA collaboration).
We also show that these methods are equally applicable to response adaptive designs that adjust the randomization probabilities in response to the observed past with a TMLE that uses the true randomization probabilities in order to be fully unbiased for any causal question of interest.
Note: This talk covers/reviews many advances that have been presented in the literature over the last decades that are deemed highly relevant for RWE studies in our active collaborations, while these advances are often not known or well understood by practitioners, but also require careful implementation and evaluation in their settings.
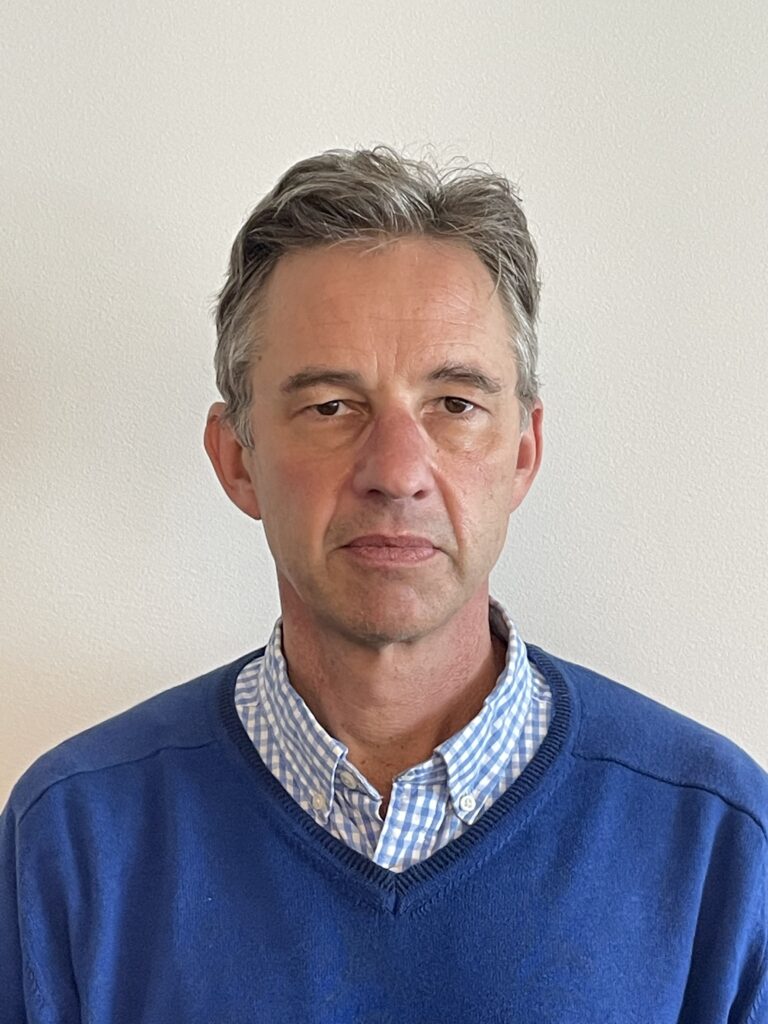
Professor Mark J. van der Laan is the Hsu/Peace Professor of Biostatistics at the University of California, Berkeley School of Public Health, and co-director of its Center of Targeted Learning and Causal Inference. He is the recipient of the 2005 COPSS Presidents’ and Snedecor Awards, as well as the 2004 Spiegelman Award, and is a Founding Editor for the International Journal of Biostatistics and the Journal of Causal Inference. His methodological research interests include censored data, causal inference, machine learning, super-learning, multiple testing, semiparametric estimation theory, Highly Adaptive Lasso, and targeted learning. He has co-authored various books, including Springer books Targeted Learning: Causal Inference for Observational and Experimental Data (2011); Targeted Learning in Data Science: Causal Inference for Complex Longitudinal Studies (2018); Multiple Testing Procedures with Application to Genomics (2008). He has graduated over 60 Ph.D students and 20 postdoctoral scholars.
More keynote speakers will be added.